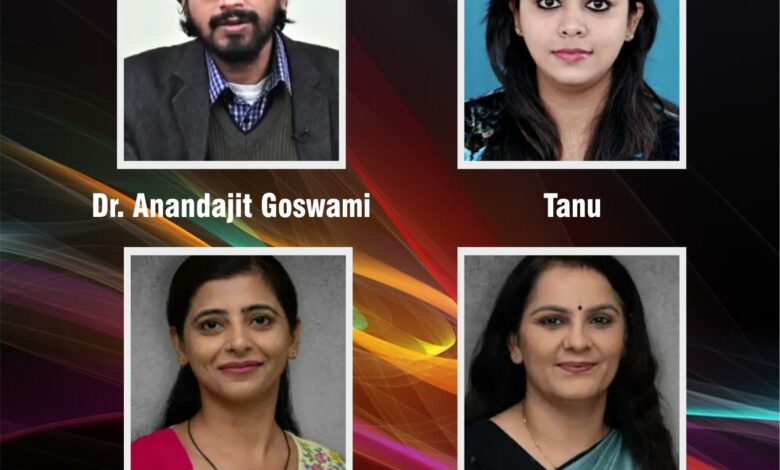
Forecasting Agricultural Futures: Machine Learning Unveils Risks and Solutions for India’s Agro-Economy Toward 2080
By Anandajit Goswami , Swati Hans , Tanu & Indu Kashyap
The economic development of agro-based nations is significantly influenced by agricultural planning. An essential factor in agricultural planning is crop choice (s). Crop selection depends on several factors, including the production rate, market prices, and governmental regulations. Numerous studies used statistical or machine learning methods to investigate crop production rate prediction, weather prediction, soil classification, and crop classification for agricultural planning. This present article applies a machine learning-based structural model to predict the nature, and degree of risks of variability in agricultural crop production in the future in the time horizon till 2080 when India aims to attain a net zero economy.
To create a net zero economy where the economy grows with the least possible carbon footprint, the agriculture sector has to be a key area of focus in the future in the time horizon of 2030 – 20700. It is therefore imperative that the agricultural sector of the Indian economy double the farmer income in a carbon-neutral way. However, the impending challenges from the human induced climate changes can pose a risk in attaining that. Changes in weather patterns, external factorsin countries like India can impact agricultural production, affect the yield, and can impact the farmer’s income in the future. Major crops like rice and wheat can be exposed to such risks of variation in the production of rice, and wheat impacting the farmers’ income, and per capita food availability.
In the literature context of India, some of the research already exists in this domain. Ekaansh Khosla, Ramesh Dharavath&RashmiPriya (2020)[1] have applied modular artificial neural networks (MNNs) to predict the amount of rainfall that can occur during the monsoon season in Visakhapatnam in the upcoming years. After predicting rain, they made a feature selection to select only essential features in predicting various kharif crops in Visakhapatnam. Finally, using only those features, they predicted the yield of bajra, maize, rice, and ragi. It can be seen from the results that in recent years less area is given to crops if we compare the area given to crops in the year 1997; this is because of the increasing population in the city as the area which was given to crops earlier has now been used as a residential area. In this study, they predicted the yield of various kharif crops in Visakhapatnam. As they have used only rainfall and area attributes in predicting the yield of crops, the yield of the specific crop depends on many other factors like population, domestic supply, area harvested, etc. If those parameters are considered, the accuracy of predictions will increase. Hence, there is still substantial space for improving the projections.Smart farming approaches include the collection of information related to crop production of various crops regionally throughout the global space by database management systems is a novel criterion in recent agricultural technology enhancement (Joshi and Kaushik., 2021)[8].
To feed the increasing population of India, there is a need to incorporate the latest technological methodologies in the agricultural sector. In addition, farmers require timely advice to predict crop productivity so that they can make proper strategies to increase the yield of their crops.
Precision agriculture is an approach that uses technology to ensure that soil and crops get what they need for optimum productivity and health. In precision agriculture, real-time farm and weather data are collected using sensors to make predictions to help farmers make correct farm-related decisions. Small sensors are deployed on the farm, collecting and sending the data to the relevant data storage node. The data collected are huge in volume and can be processed using big data analytics. Big data provides facilities like data storage, data processing, and data analysis with accuracy. So its use in agriculture can benefit farmers and the nation’s economic growth. With the help of big data analytics and related machine learning algorithms, crop productivity can be increased by many folds. To accommodate the prediction strategies for Kharif, they have presented a methodology named MANNs-SVR[1] in this study. By using MANNs-SVR[1], first, they predicted the occurrence of rainfall in the region of Visakhapatnam. Then, by using attributes that give the most information about the production of Kharif crops in Visakhapatnam, they predicted the amount of common kharif crops, i.e., rice, ragi, maize, and bajra, that can be yielded in the upcoming years. Most past studies mainly focused on image processing and prediction using statistical models. However, we have proposed to use machine learning approaches that are faster in computation and more efficient than statistical methods proposed in the past.
Shank et al. (2008)[9] used weather data from twenty locations in Georgia, United States, to construct an ANN to predict various freezing conditions and heat wave occurrences, which can affect crop production. Therefore, it can be stated that ANN models have the potential to predict additional information about the cropping system and management. It is useful for the prognostication of different meteorological variables for the prediction of better agriculture yield in the future.In this research and study, machine learning algorithms have beenexplored to design an efficient system for accurate crop yield forecasting.
Varalakshmi, T.,[10] et al. discovered that the usage of a deep-learning model forrainfall prediction using LSTM in agriculture canimprove the accuracy of model predictions.
LSTM networks are well suited for the classification, processing, and prediction of time series data because there may be delays of unknown duration between important events in a time series. Their results show that these deep-learning models can predict weather features accurately enough to compete with traditional models.
Bhardwaj, Mayank Ratan,[11] et al. proposed an innovative deep learning-based approach to achieve increased accuracy in the price prediction of crops. The proposed approach uses graph neural networks (GNNs) in conjunction with a standard convolutional neural network (CNN) model to exploit geospatial dependencies in the prices of crops. This method performs at least 20% better than the results seen in the literature while working effectively with noisy legacy data.
Anuradha, T.,[12] et al. aim to predict future crop yields accurately using a machine learning model.The model uses data on crop production in different districts and years to train and test various machine learning (ML) Techniques like decision trees, linear regression, KNN clustering (nearest neighbors) along with a deep learning architecture. They found that to predict crop yields, the KNN model can be preferred over linear regression and decision tree. Among the three models, the KNN model has the lowest RMSE and MAE values, indicating that it is the most accurate and reliable model for predicting crop yields. The LSTM architecture model also
increased the accuracy and had less error rate while we performed separately. LSTM architecture indicated to be the best compared with those three machine learning models.
Ramya, Mrs P.,[13] et al. proposed a model created by using the deep learning (RNN) technique. They also implemented some other algorithms to compare the accuracy of prediction. The model predicts the best crops that should be grown on land with fewer expenses among several crops available after analysing the prediction parameters.
From the existing literature, it is evident that climate variables, and external factors like land use, do impact agriculture yields in the future. This thereby affects the livelihood and security of farmers in the future. Therefore, the importance of LSTM approaches in understanding the vulnerability of farmers to future climate vulnerability scenarios is scientifically proven across the developing country context. Hence for a country like Indiawith a large scale dependence of population(around 74%) on agricultural yields for the future, the relevance of the LSTM approach in predicting the agricultural risk for farmers acrossvarious external scenarios increases manifold.Moreover, a machine learning-based LSTM model can facilitate efficient, effective agricultural policy-making for the future by understanding the nature and degree of agricultural risk across the states of India.Hence, the below-mentioned methodology (as shown in Exhibit 1) of prediction is used in the present analysis –
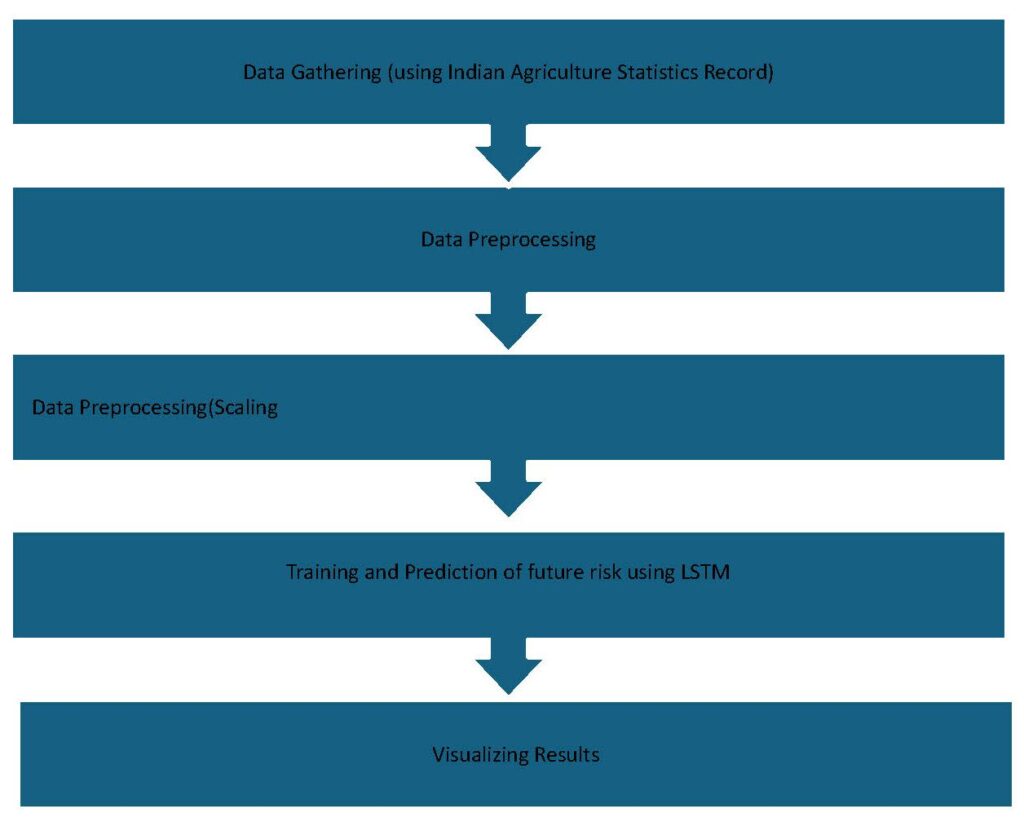
In this methodology,the dataset is gathered for the years 1961-2020 and is fed to the LSTM model to forecast the riceand wheat production and imports for the years 2021-2080. Before training the model, data is pre-processed by removing null values and scaling data. In the LSTM model, previous five-year data is used to predict sixth-year data. After predictions, results of production and import of both rice and wheat are visualized for the years 1961-2080
Using the above methodology with the LSTM model, the predictions for rice and wheat productions in tonnes per capita for India are forecasted for upcoming years till 2080 as shown below-
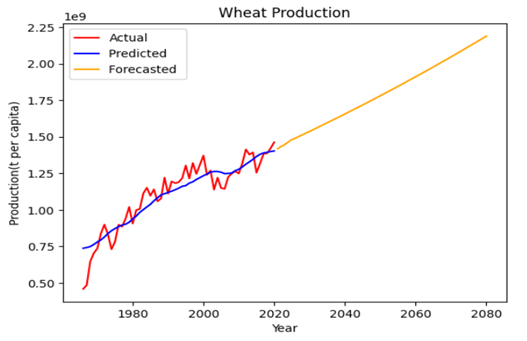
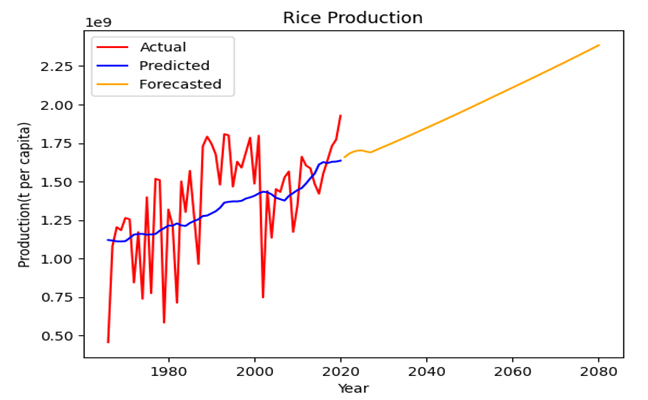
The predictions for wheat and rice imports in tonnes per capita for India are forecasted for upcoming years till 2080 –
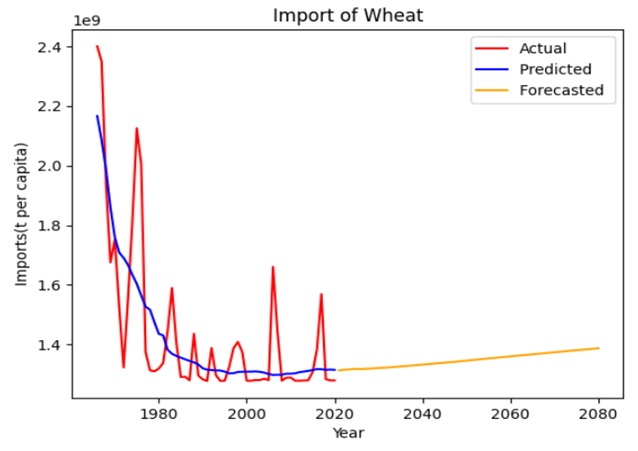
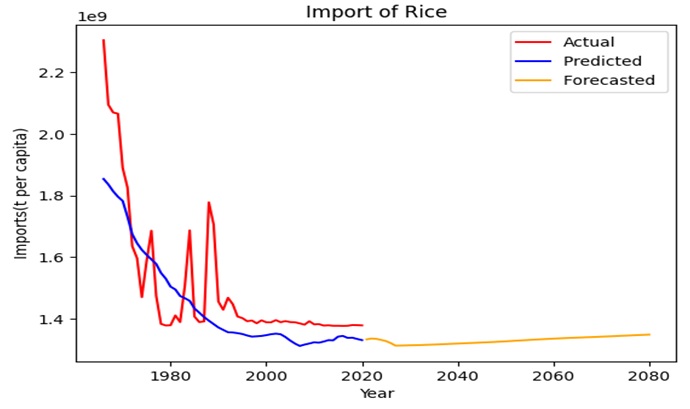
Our analysis uses the variations in population, land usage, area harvested, production, yield, and domestic supply(tonnes per capita) as input variables. All these input variables can change due to the impacts of human-induced climate change. Using the above as input variables, the model predicts the rice and wheat production (tonnes per capita) which caters to the staple diet of the majority of the Indian population across the States of India.
Our analysis uses a Machine Learning model by integrating changes in above above-mentioned input variables. The model is further used to predict the future rice and wheat imports (tonnes per capita) in India. The modeling results indicate that rice and wheat production in India doesn’t increase exponentially or in tandem with a rise in population. Further imports of rice and wheat increase in the future in India in the time frame till 2070. This highlights that climate vulnerability exposure to Indian agriculture raises food security risks for the larger population as the production increase in rice and wheat doesn’t match with a comparable rise in population increase which are dependent on rice and wheat for their staple food.
Moreover, to match the gap in the demand and supply of rice and wheat, the imports of rice and wheat do not increase to a substantial amount to meet this gap.
This hints towards urgent policy measures to enhance our food sufficiency against the backdrop of the advent of adverse impacts of climate change in the future. Further, it also enhances the importance of creating a switchover to alternative food crops for nourishment, and nutrient requirement. This can come from the larger population mostly from millets which have a higher nutrient value and are less water-consuming. Further, the Indian food diet system has to switch to alternative food systems that are less exposed to the vagaries of variation in weather, rainfall, and other natural resource-dependent factors.
If India has to be food self-sufficient, double the farmer income, and at the same time attain a carbon-neutral net-zero economy even amidst the adverse effects of climate change, the time has come to take some emergency actions to make the country self-reliant on alternative, sustainable food systems for future.
References:
- Khosla, E., Dharavath, R. and Priya, R., (2020). Crop yield prediction using aggregated rainfall-based modular artificial neural networks and support vector regression. Environment, Development and Sustainability, 22, pp.5687-5708.
- Kang, Y., Khan, S., & Ma, X. (2009). Climate change impacts on crop yield, crop water productivity and food security—A review. Progress in Natural Science, 19(12), 1665–1674.
- Kannan, E., & Sundaram, S. (2011). Analysis of trends in India’s Agricultural Growth. Bangalore: Institute for Social and Economic Change.
- Kumar, A., &Bhattachrya, S. (2015). Crop yield prediction using Agro Algorithm in Hadoop. International Journal of Computer Science and Information Technology & Security (IJCSITS), 5(2), 271–274.
- Mo, X., Liu, S., Lin, Z., Xu, Y., Xiang, Y., & McVicar, T. R. (2005). Prediction of crop yield, water consumption, and water use efficiency with a SVAT-crop growth model using remotely sensed data on the North China Plain. Ecological Modelling, 183(2), 301–322.
- Moriondo, M., Giannakopoulos, C., & Bindi, M. (2011). Climate change impact assessment: The role of climate extremes in crop yield simulation. Climatic Change, 104(3–4), 679–701.
- Panda, S. S., Ames, D. P., & Panigrahi, S. (2010). Application of vegetation indices for agricultural crop yield prediction using neural network techniques. Remote Sensing, 2(3), 673–696.
- Joshi, A., Kaushik, V., 2021. Big Data and its analytics in agriculture. Bioinformatics for Agriculture: High-throughput Approaches. Springer, Singapore, pp. 71–83.
- Shank,D.B.,Hoogenboom,G.,McClendon,R.W.,2008. Dewpoint Temperature Prediction using Artificial Neural networks.J.Appl.Meteorol.Climatol.47(6),1757–1769.
- T. Varalakshmi, SK. Nazeer, V. Srimanth, S. Naga sai Krishna, SK. Sahool, Downpour Forecasting Using Deep Learning.Journal of Engineering Sciences,Vol 14 Issue 03,2023
- Bhardwaj, Mayank Ratan, et al. “An innovative Deep Learning Based Approach for Accurate Agricultural Crop Price Prediction.” arXiv preprint arXiv:2304.09761 (2023).
- Anuradha, T., et al. “Estimating Crop Yields Using Machine Learning Techniques.” Journal of Survey in Fisheries Sciences 10.2S (2023): 2778-2782.
- Ramya, Mrs P., et al. “CROP YIELD PREDICTION USING RECURRENT NEURAL NETWORK.”, International Research Journal of Modernization in Engineering Technology and Science, Volume:05, March-2023:476-480
- Our World of Data, https://ourworldindata.org/agricultural-production#introduction
Disclaimer: All Views Are Personal